Logistic regression python code with example
Learn logistic regression python code with example. The logistic regression is used for predicting the binary categorical variable means those response variables which have only 2 options. They can be used to identify the person is diabetic or not and similar cause. The logistic regression is a special case of a linear regression model and response variable is binomial categorical. If you are continuous or discrete data and looking to do prediction you apply linear regression. And if you have categorical data but more than two classes you apply a decision tree or random forest algorithms. The first condition for logistic regression in python is the response variable should be a categorical variable. And binomial categorical variable means it should have only two values- 1/0. If we have two value in the form of Yes/No or True/False, first convert it into 1/0 form and then start with creating logistic regression in python.
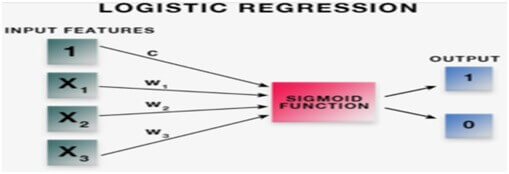
Logistic regression
Logistic Regression is a Machine Learning algorithm and used to predict the probability of a categorical dependent variable.
The dependent variable is a binary variable that contains data coded as 1 or 0.
In logistic Regression the Regression is a supervised machine learning algorithm that is used in binary classification.
It is binary because one of the limitations of Logistic Regression that it can categorize data with two distinct classes.
The regression technique is dependent variable is categorical. Let us look at an example,
Where we are trying to predict whether it is going to rain or not based on the independent variables: temperature and humidity.
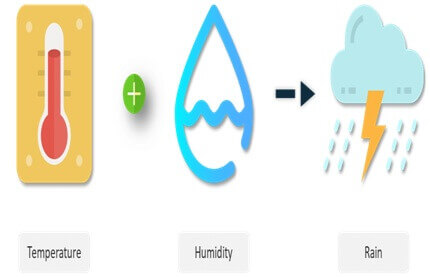
The Logistic Regression is a supervised as machine learning algorithm used in binary classification.
We say it binary
because one of the limitations of logistic Regression .It is the fact that it can only categorize data with
two distinct classes.
The Logistic Regression will fit in a line to a dataset and returns the probability that a new sample belongs to one of the two classes
It
is a Machine Learning classification algorithm that is used to predict the probability of a categorical dependent variable.
The logistic regression model P(Y=1) is as a function of X.
Logistic Regression Assumptions:-
- The binary logistic regression requires the dependent variable to be binary.
- For binary regression the factor level 1 of the dependent variable should represent the desired outcome.
- The meaningful variables should be included in the logistic regression.
- The independent variables should be independent of each other in logistic regression.
- The model should have little and no multicollinearity.
- The variables are linearly related to the log odd and will require quite large sample sizes.
It is a technique to analyze a data-set which has a dependent variable and one or more independent variables to predict the outcome in a binary variable means it will have two outcomes.
The dependent variable is categorical in nature.
Dependent variable referred as target variable and the independent variables are called the predictors.
Logistic regression is a case of linear regression where we only predict the outcome in a categorical variable.
We use the sigmoid function to predict the categorical value and threshold value decides the outcome.
Linear regression equation is written as follows:
y = β0 + β1X1 + β2X2 …. + βnXn
- Y stands for the dependent variable that needs to be predicted.
- β0 is the Y-intercept is basically the point on the line which touch the y-axis.
- β1 is the slope of the line and X here represent the independent variable that is used to predict our resultant dependent value.
Sigmoid function: p = 1 / 1 + e-y
Apply sigmoid function on the linear regression equation.
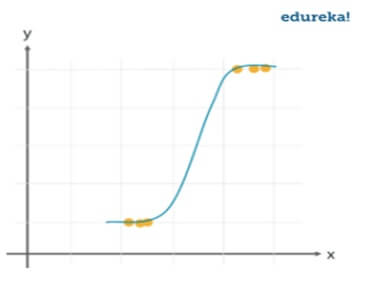
Logistic Regression equation:
p = 1 / 1 + e-(β0 + β1X1 + β2X2 …. + βnXn)
Take a look at different types of logistic regression.
Types of Logistic Regression:-
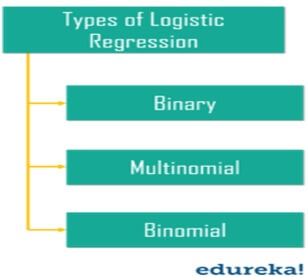
Logistic Regression is a classification of algorithm used to predict the probability of a categorical dependent variable.
The log Logistic regression is a popular method to predict a categorical response.
It is a special case of generalized linear models that predicts the probability of the outcomes.
Use the parameter to select between these two algorithms or leave it unset and Spark will infer the correct variant.
The multinomial logistic regression is used for binary classification by setting the family param to “multinomial”.
Logistic regression will produce two sets of coefficients and two intercepts.
After fitting the logistic regression Model the intercept on dataset with constant nonzero column, Spark MLlib outputs zero coefficients for constant nonzero columns.
Logistic regression Advantages : -
- The logistic regression will perform dataset linearly and separable.
- The regression is less prone to over fitting but convert over fit in high dimensional datasets.
- You should consider regularization technique to avoid over fitting in these scenarios.
- Logistic regression not only gives measure of how relevant a predictor is but also its direction of association is positive or negative.
- It is easier to implement, interpret and very efficient to train all.
Logistic regression Disadvantages: -
- The main limitation is assumption of linearity between dependent variable and independent variable. In real world data is linearly separable and most of time data could be jumbled mess.
- If the number of observation are less than number of features should not be used it may lead to overfit.
- The logistic regression can be used to predict discrete functions and dependent variables are restricted to district number set.
- This restriction is problematic as it is prohibitive to prediction of continuous data.